

Then we describe our experimental data and the evaluation method (Section 3), after which we proceed to describe the various author profiling strategies that we investigated (Section 4). In the following sections, we first present some previous work on gender recognition (Section 2). For all techniques and features, we ran the same 5-fold cross-validation experiments in order to determine how well they could be used to distinguish between male and female authors of tweets. We also varied the recognition features provided to the techniques, using both character and token n-grams. 2004), with and without preprocessing the input vectors with Principal Component Analysis (PCA (Pearson 1901) (Hotelling 1933)). We then experimented with several author profiling techniques, namely Support Vector Regression (as provided by LIBSVM (Chang and Lin 2011)), Linguistic Profiling (LP (van Halteren 2004)), and TiMBL (Daelemans et al. For our experiment, we selected 600 authors for whom we were able to determine with a high degree of certainty a) that they were human individuals and b) what gender they were. In this paper, we start modestly, by attempting to derive just the gender of the authors1 automatically, purely on the basis of the content of their tweets, using author profiling techniques.
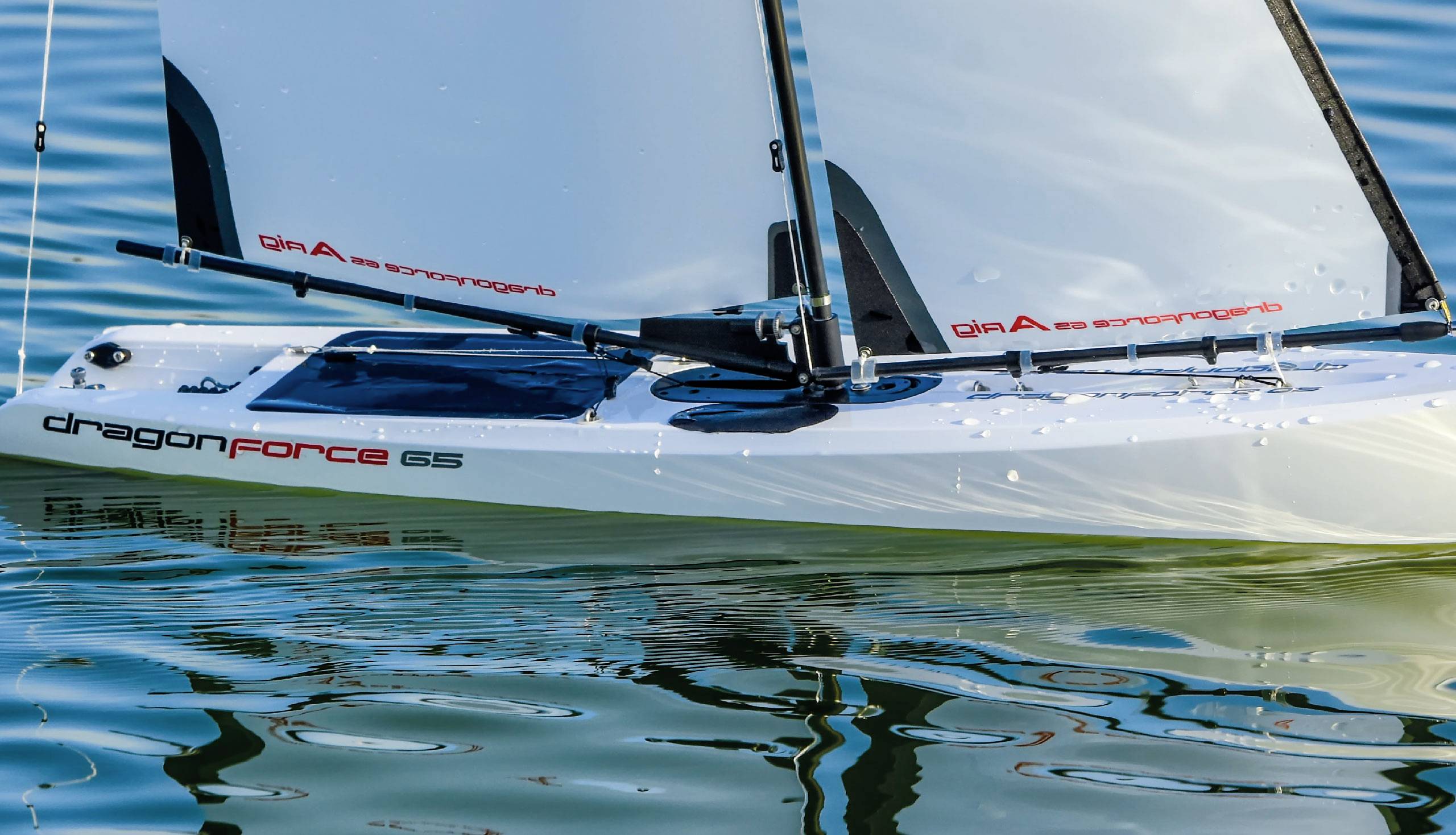
The resource would become even more useful if we could deduce complete and correct metadata from the various available information sources, such as the provided metadata, user relations, profile photos, and the text of the tweets. And, obviously, it is unknown to which degree the information that is present is true. In this case, the Twitter profiles of the authors are available, but these consist of freeform text rather than fixed information fields. However, as any collection that is harvested automatically, its usability is reduced by a lack of reliable metadata. Introduction In the Netherlands, we have a rather unique resource in the form of the TwiNL data set: a daily updated collection that probably contains at least 30% of the Dutch public tweet production since 2011 (Tjong Kim Sang and van den Bosch 2013). Two other machine learning systems, Linguistic Profiling and TiMBL, come close to this result, at least when the input is first preprocessed with PCA.ġ. We achieved the best results, 95.5% correct assignment in a 5-fold cross-validation on our corpus, with Support Vector Regression on all token unigrams. We experimented with several authorship profiling techniques and various recognition features, using Tweet text only, in order to determine how well they could distinguish between male and female authors of Tweets.
OVERLOOP ZEILBOOT FULL
Radboud University Nijmegen, CLS, LinguisticsĪbstract In this paper, we investigate gender recognition on Dutch Twitter material, using a corpus consisting of the full Tweet production (as far as present in the TwiNL data set) of 600 users (known to be human individuals) over 20. Gender Recognition on Dutch Tweets Hans van Halteren Nander Speerstra Computational Linguistics in the Netherlands Journal 4 (2014) 171-190
